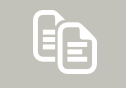
Collection of human reaction times and supporting health related data for analysis of cognitive and physical performance
Smoking, excessive drinking, overeating and physical inactivity are well-established risk factors decreasing human physical performance. Moreover, epidemiological work has identified modifiable lifestyle factors, such as poor diet and physical and cognitive inactivity that are associated with the risk of reduced cognitive performance. Definition, collection and annotation of human reaction times and suitable health related data and metadata provides researchers with a necessary source for further analysis of human physical and cognitive performance. The collection of human reaction times and supporting health related data was obtained from two groups comprising together 349 people of all ages - the visitors of the Days of Science and Technology 2016 held on the Pilsen central square and members of the Mensa Czech Republic visiting the neuroinformatics lab at the University of West Bohemia. Each provided dataset contains a complete or partial set of data obtained from the following measurements: hands and legs reaction times, color vision, spirometry, electrocardiography, blood pressure, blood glucose, body proportions and flexibility. It also provides a sufficient set of metadata (age, gender and summary of the participant\'s current life style and health) to allow researchers to perform further analysis. This article has two main aims. The first aim is to provide a well annotated collection of human reaction times and health related data that is suitable for further analysis of lifestyle and human cognitive and physical performance. This data collection is complemented with a preliminarily statistical evaluation. The second aim is to present a procedure of efficient acquisition of human reaction times and supporting health related data in non-lab and lab conditions.